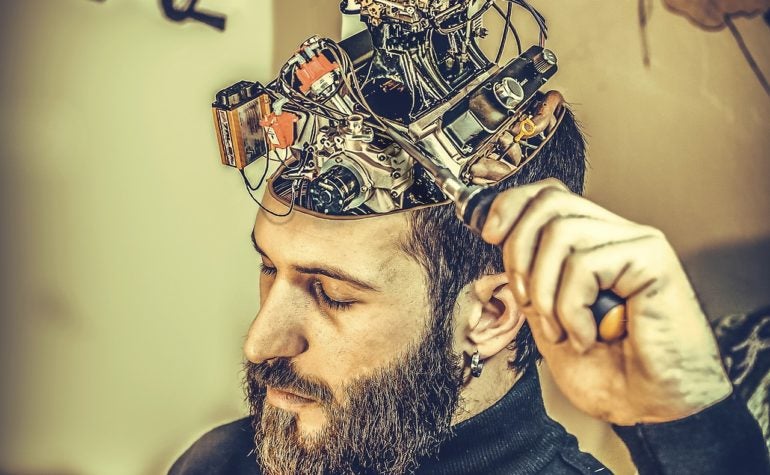
Artificial intelligence (AI) has been advancing at phenomenal speeds on many fronts, but it’s also beset by challenges in a few key areas. Can edge computing help?
Ed Nelson, director of the AI Hardware Summit and Edge AI Summit, helps us understand a few of the challenges facing artificial intelligence and then explains how edge computing can help resolve them.
Editor’s Note: This is a guest post from an industry expert. The State of the Edge blog welcomes diverse opinions from industry practitioners, analysts, and researchers, highlighting thought leadership in all areas of edge computing and adjacent technologies. If you’d like to propose an article, please see our Submission Guidelines.
AI has Outgrown the Traditional Cloud Paradigm
In May, OpenAI reported that the amount of compute needed to process the largest deep learning training runs is doubling roughly every 3.5 months. As the semiconductor industry wrangles with the challenges of moving to 7 nanometer nodes, and Moore’s Law looks to be all but coming to its logical and physical conclusion, the requirements for computational power continue to increase exponentially. In the main, the development of computationally-intensive and sophisticated algorithms has been outpacing the advancements of hardware—and this trend shows no signs of abating.
In the data center, where the majority of computational resources are located, and where all but a few machine learning models are trained, AI’s insatiable thirst for computation brings its own unique set of logistical challenges. How to maximize throughput while minimizing power consumption, how to disperse heat, how to transfer data between processor and memory, and how to reduce latency, to name a few. In the last 24 months, many of the largest global cloud providers have announced custom-built processing units for AI training and inference, as the semiconductor industry gets a much-needed injection of innovation and growth from this burgeoning market. On top of this, at least 45 new AI chip startups have appeared across the globe in an effort to be the first to reap the rewards of increasing demands for AI.
Issues of data transfer further compound the challenges of inadequate hardware. Use cases for machine learning are emerging that cannot afford to be constrained by power consumption, bandwidth, latency, connectivity and security issues. The self-driving car offers a use case that reflects all of these concerns. An autonomous vehicle needs to make life-or-death decisions in a time-critical manner, regardless of whether cloud connectivity exists or not, and the data contained within the car needs to be totally secure. Additionally, inferencing done on board the car needs to be carried out at extremely low power so that the majority of the car’s energy can be used for its primary purpose —getting its passengers from A to B. Currently, with computational resources located primarily in centrally-located cloud data centers, and latency and connectivity issues being far from resolved, the conditions simply do not exist wherein an (almost) totally reliable, safe and energy-efficient autonomous vehicle can operate.
The Edge can Help Resolve the Challenge
All is not lost. The world of edge computing offers compelling solutions to these challenges. Chip companies, cloud providers, edge infrastructure companies, IoT-invested enterprises and AI solutions developers are increasingly focused on delivering the “Intelligent Edge”, a computing paradigm that will unleash a wave of new markets and business models and make AI truly ubiquitous.
The Intelligent Edge is not a particularly revolutionary term and is defined in a variety of ways by a variety of people and institutions. In the light of the recent State of the Edge report, I will posit my own summary of what this ecosystem may look like.
The Intelligent Edge will take the form of a new decentralized internet paradigm, wherein computational resources, and thus AI workloads, are distributed more evenly between the centralized cloud and the edge of the network. Machine learning-enabled devices at the device edge will handle low-level AI tasks, supported by micro data centers and edge computing nodes positioned at the infrastructure edge, which will be geographically and logically close to devices, and will be capable of handling much larger data sets and much more complex workloads. Thus, complex AI workloads that cannot be processed on the device will be handed off to cloud workloads at the edge.Workloads of a less urgent nature, or of larger scope, will be split between the cloud resources at the infrastructure edge and the much larger resources of the centralized cloud.
By deploying micro data centers and edge computing nodes with the latest AI processing capabilities,, we shorten the distance between the collection of data and its cloud processing. Rather than devices shunting all of their data to the centralized cloud for processing, and the cloud shunting it back (at great cost in time, money and security), the Intelligent Edge will allow for certain time-critical and security-sensitive AI applications to operate either entirely on a device, or in conjunction with localized data centers, vastly reducing latency, bandwidth requirements, power consumption and cost, while improving security and privacy.
How might that work?
In the case of our autonomous car, the Intelligent Edge would enable a single vehicle to identify a pothole in a road in Boston, for example, and take the following actions:
1. On-Device (Device Edge): Identify the pothole via AI inference and make the necessary adjustments to avoid it.
2. Local Micro Data Center (Infrastructure Edge, Edge Cloud): Communicate to a local data center the location of the pothole, its specifications and the timing that it was spotted, so that cars in the area of Boston may be alerted to its presence. If any more complex decision making needs to be done and communicated to the car in question, or several in the region, it can be done here.
3. Centralized Cloud: Communicate metadata to the Cloud that may be stored in a database of national significance or utilized in future training scenarios. Any decision-making that might need to be done that includes huge numbers of parameters (our pothole being one) and affects thousands of cars nationwide could be done here.
Let’s build the Intelligent Edge!
Many people are already building the Intelligent Edge. Advancements at the device edge predominantly focus on hardware and ever-smaller intelligent processing units that run at very low power. Software developers are migrating existing AI workloads towards the edge, and developing edge-native AI applications optimized for edge environments.
At the infrastructure edge, several companies are rolling out massive international micro data center deployments, many working with the telcos to distribute edge computing nodes throughout the world. As telcos upgrade their networks to support 5G, they will also be in a position to deliver the infrastructure needed to realize the Intelligent Edge. Between the edge devices, the infrastructure edge and the centralized cloud, there is a vibrant ecosystem of companies focused on connecting, securing and optimizing the edge.
Bringing forth this new internet infrastructure will require equal attention to both the device edge and the infrastructure edge, which are significant and difficult tasks. But a number of companies have taken up this task and have identified the benefits of building the edge and making it intelligent. The pursuit of this goal represents nothing less than a wave of opportunity; firstly, to reduce the pressure on today’s cloud and move away from centralized computing; secondly, to solve many of the issues that we face in artificial intelligence R&D; finally, to unleash a new generation of services, applications and business models that are enabled by AI at the edge of the network.
Ed Nelson is a conference producer at Kisaco Research, a London-based commercial events company that executes industry-leading conferences in the technology, pharmaceutical and consumer lifestyle industries, among others. He has held several roles spanning from software design analysis to reservist military service. Ed heads up KR’s technology portfolio, which has historically covered Robotic Process Automation & Digital Transformation, and now includes AI Hardware and Edge AI. He holds a degree in History from Newcastle University, and a postgraduate degree from the University of Leeds.
Please consider attending one or more of Ed’s upcoming conferences, including the AI Hardware Summit, September 18-19, 2018, Computer History Museum, Mountain View, CA and the Edge AI Summit, December 11, 2018, San Francisco, CA.
Opinions expressed in this article do not necessarily reflect the opinions of any person or entity other than the author.